Stefan Leichenauer, VP of Engineering at SandboxAQ, has penned an exclusive op-ed for April’s edition of CNME, where he makes the case that businesses seeking success with AI, need to look beyond LLMs, and instead focus on LQMs.
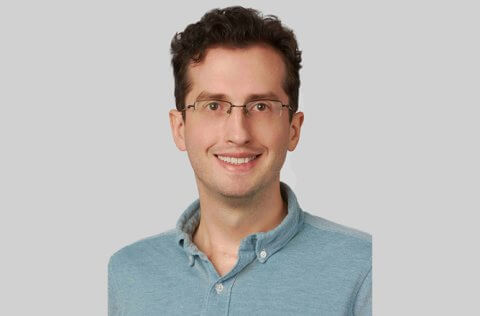
The Middle East has for a while now signaled its intentions of being a leading player in the AI revolution. And while the United Arab Emirates (UAE) became the first nation globally to appoint a Minister of Artificial Intelligence back in 2017, it was the advent of generative AI that has truly accelerated the region’s ambitions. In a study of 63 use cases, McKinsey estimated the economic potential for GenAI in the GCC: it could be responsible for between US$21 billion and US$35 billion of annual value, to accompany a projected US$150 billion generated by other AI technologies in the region.
But the large-language models (LLMs) that form the foundation of many, if not most, regional GenAI solutions are just the start of the story. Already another form of machine learning is getting onto the radar of forward-focused decision makers around the globe — large quantitative models (LQMs).
While LLMs work with and generate language content, LQMs do the same with quantitative data that is ordinarily handled through purely numerical methods.
The challenges we face in areas such as finance, healthcare, and sustainability are not fundamentally language-based challenges, and so the most effective and natural tools are not language-based tools. For a region that has shown laudable ambition in these areas, LQMs will be transformative.
On the back of the UAE’s’ recent hosting of COP28, LQMs could help with net-zero initiatives by optimizing renewable energy integration and improving climate modelling to guide sustainable policies. And as Gulf nations ramp up their manufacturing sector to boost non-oil GDP, they can ensure businesses have access to the best material science through LQMs’ ability to accelerate R&D. We’re already seeing industry leaders taking action, with Aramco recently revealing its strategy for leveraging quantitative AI models for increasing value of downstream products.
The right tool for the job
To be clear, LQMs are not here to replace LLMs. In fact, in many cases the tools are complementary. While LLMs are fantastic for human-machine-interaction, the imprecise nature of language means that when acting alone, they will not solve quantitative problems. For example, while a user-facing LLM may work well for basic software development, additional heuristic layers may be required to align with best practices and compliance obligations. Also, an LLM may be great for customer service, but it would be an inappropriate tool for, say, pharmaceutical drug discovery.
Where precision is paramount, LQMs are indispensable. Let’s say you are trying to predict the weather. You have data from the past three days, and you want to predict the weather for the next three days. Imagine putting an LLM to work on the task. It acts as a sophisticated almanac, and having trawled through thousands of weather reports will extrapolate patterns, potentially delivering a result that’s accurate most of the time. However, if billions of dollars or thousands of lives are on the line, “most of the time” simply isn’t enough. In predicting the next hurricane, the next wildfire, or the next sandstorm, LQMs would include physics-based models, AI models, and data from past events — any relevant technique to encode the underlying phenomena of the weather, leading to reliable and trustworthy predictions. This approach is a far cry from the simple LLM-based approach, and clearly more aligned with the task.
LLMs consume the data they’re served. LQMs create their own meals. The capabilities of an LLM are determined by the data it was trained on, which in most cases is just everything on the open web. There is no great store of data beyond that, and that is a limitation that LLMs have to deal with. With an LQM, we can always generate trustworthy data because we understand the fundamental principles underlying the data. We have the equations, and we can solve them in a new domain to give our models something new to train on. This infinite extensibility is special to LQMs, and is part of why they have the ability to go well beyond what LLMs alone can do.
Yes, LLMs are excellent at conversation and search and can even take bold initiatives in certain controlled circumstances where they have been optimized for a specific task. But they cannot match LQMs for their ability to optimize for case-specific objectives and parameters. For example, in battery development, where lithium-ion technology has dominated for 45 years, LQMs can correct the stall in progress by simulating millions of possible chemical combinations without the need for physical prototyping. Such models can also be of great help in pharmaceutical development, where traditional approaches are beset with the high failure rates of clinical trials. LQMs can analyze molecular structures and interactions at the electron level, drastically reducing the costs of trial phases.
Arts versus science
Drug discovery, materials science, healthcare diagnostics, financial modelling, and industrial optimization — these are all growth areas in the GCC. Since the region’s governments launched their national AI strategies, the field has expanded rapidly, culminating in the rise of GenAI. But the challenges of the day will call for decision makers to dig deeper into the AI toolbox. The precision and data-driven insights provided by LQMs are just such a tool.
LQMs also operate by reaching for the most appropriate tool (be it GenAI, non-GenAI, or even non-AI) or data to solve the problem at hand. This flexibility makes LQMs an ideal investment for regional enterprises that have sought to solve their complex problems with LLMs alone, only to be disappointed. That’s why forward-thinking leaders are exploring LQMs as a critical tool for tackling complex, quantitative challenges.